A New Artificial Intelligence Model for a More Accurate Lung Cancer Diagnosis
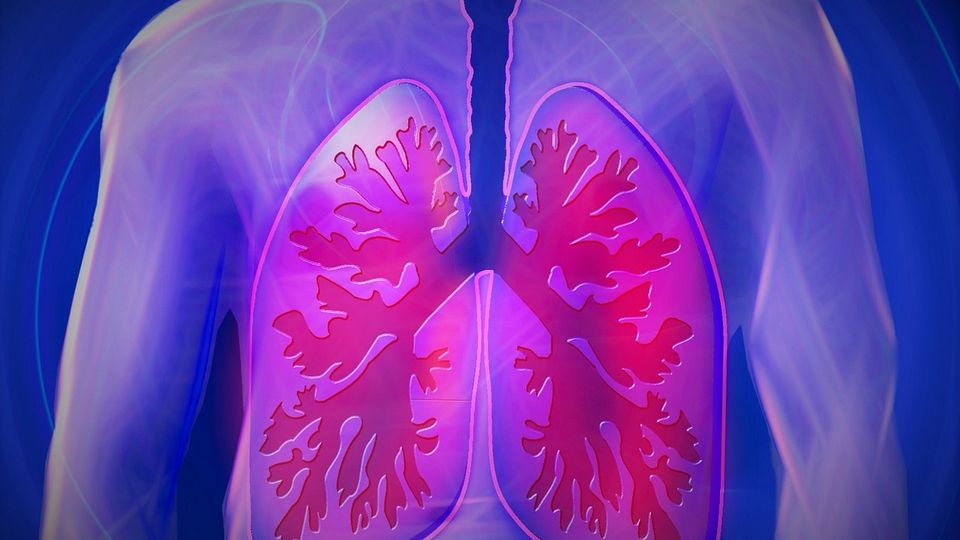
Lung cancer is one of the most lethal cancers in the United States, witha five-year survival rate of only 18.6 percent. The sources of this leading cause of cancer-related deaths includeoccupational exposure to toxic substances,如石棉。即使在石棉暴露has ceased, a prior persistent and long-term exposure in the workplace can contribute to the disease's evolution.
The survival rate for lung cancer may be poor because, often, doctors find it late when it is already difficult to treat. At the moment, medical professionals diagnose84 percentof lung cancer cases at an advanced stage. The prognosis for lung cancer is substantially better when lung cancer is discovered early by radiologists.
Artificial intelligence (AI) models for timely detection could help to save many people. AI programs are already discovering subtle patterns in computed tomography (CT) scans of patients and can be more accurate at lung nodule detection than trained radiologists.
AI models detected malignant nodules more accurately than trained radiologists
Lung cancer is diagnosed by examining a sample of lung cells in the laboratory. However, some lung cancers can be found by screening, which means testing for the disease in patients with no symptoms or history.
The only lung cancer screening test that is recommended is low-dose CT. Using these CT images, medical professionals can identify the pulmonary nodules associated with the disease. However, the number of radiologists who evaluate lung images is not keeping up with demand. Overworked radiologists may make mistakes as a result of their heavy workload.
AI technologies can assist by taking the load off overworked doctors and identifying lung spots that aren't apparent to the human eye.
Research published in the journalRadiologyfound that an AI program for lung nodule assessment using deep learning (DL) could identify specific patterns in imaging data and deliver excellent results. In the research, experts from the Netherlands used CT scans of more than 16,000 nodules from the National Lung Cancer Screening Trial to instruct a DL algorithm to anticipate the malignancy risk of lung nodules. The scans were not only recent but also from before the patients were diagnosed with lung cancer.
The algorithm outperformedThe PanCan model, which forecasts the risk of nodule malignancy. The program could identify and measure cancers in CT scans exceptionally accurately, with performance comparable to thoracic radiologists.
The model will be enhanced by adding clinical aspects such as age, sex and smoking history. When AI algorithms are adequately verified, they may one day become an integral part of lung cancer screening.
In another study, an AI system also found nodules in scans of patients more accurately than trained radiologists did. This system similarly relied on the DL approach to identify lung nodules on CT scans.
The researchers used a library of over 40,000 CT scans to train the algorithm. Over time, the computer learned which visual attributes distinguish malignant from benign patches, and it improved its ability to detect early cancer indications. It outperformed a panel of six seasoned radiologists in detecting early stages of lung cancer 94 percent of the time.
New possibilities in lung cancer screening
Misdiagnosis of lung cancer on CT is caused by a number of reasons, many of which are extremely similar. According to a study, radiologist observer error is the most significant cause of misdiagnosis. The errors refer to scanning, recognition, decision-making and satisfaction of search.
In the end, the result is that radiologists often cannot precisely identify malignant nodules. Even if they already employ computer-aided diagnostic methods to aid in the detection of malignant nodules, this wastes a lot of time. The system is frequently instructed by a human programmer on what features to look for. Despite this, the computers identify many suspected cancers that are benign, requiring radiologists to evaluate each one individually.
As mentioned earlier, the need for early detection has fueled the development of AI programs capable of detecting and measuring cancer cells more effectively. These AI systems are based on DL. DL systems use real-world samples to find out what a nodule is. Researchers supply a vast data set to the algorithms, which comprises hundreds of CT scans of people's lungs, some with cancer and others without. As a result, the machines can see what a lung cancer nodule looks like for themselves.
The more training images the system sees, the better it can tell the difference between lung cancers and benign splotches. They do it with greater precision than older, non-AI systems. Some DL algorithms also provide clinicians with an assessment of their confidence level in their judgment, which can help them make better therapeutic decisions.
Screening accuracy is also improved by the capacity of a system to assess a complete 3D CT scan rather than just a series of 2D slices. Furthermore, 3D scans provide more diagnostic information about features outside of the underlying tumor, such as arteries.
AI makes significant progress
With AI performing some hard work, lung cancer screening programs may save many lives—at a lower cost—without putting as much strain on radiologists. Therefore, potential advantages of the AI models include lowering the number of unnecessary diagnostic treatments, the expense of lung cancer screening and radiologists' workload.
The AI-based assessment models will benefit patients, their families and carers. Despite its infancy, this model can play a significant role in the future of lung cancer diagnosis, contributing to the growing body of evidence supporting the use of AI in global medical advancements, opening the way for clinical trials of new treatments.
AI is set to enter a critical new phase in radiology, thanks to a growing body of research. Researchers have so far worked on how to identify specific problems before building, training, testing and validating AI models in different populations.
Now begins the most crucial step, which is still in its early stages worldwide: thorough evaluation once the model has been clinically implemented. This assessment should include tried-and-true approaches like the sensitivity rate for detecting cancers and the percentage of false-positive results.