Why Pharma Is Rethinking Its Data Integration Strategy – the Criticality of Data Integration
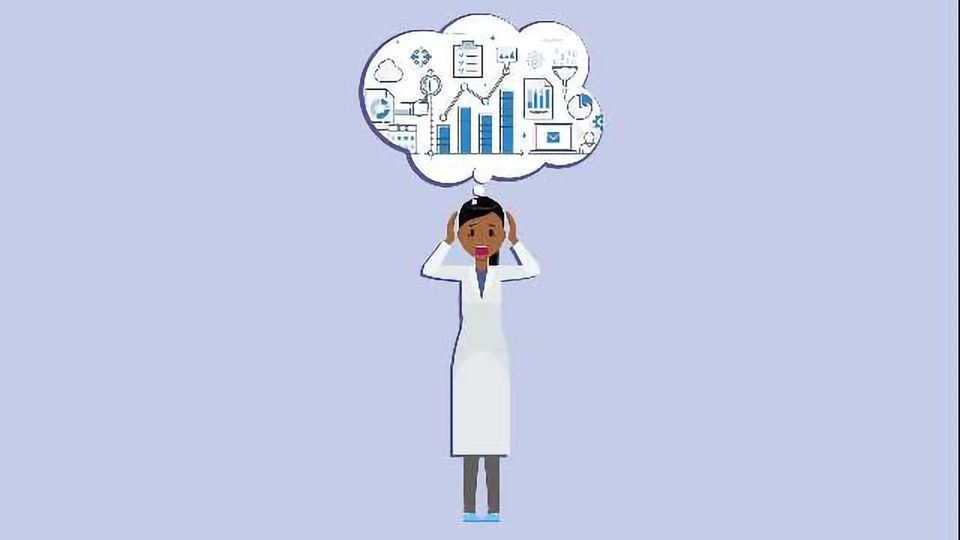
The pharma industry is being disrupted in multiple ways. M&As and technology transformations are just some of the paradigm shifts redefining healthcare strategies today. While pre-competitive collaboration initiatives are driving leaner processes, the costs of drug development are only increasing year-on-year. A precipitous need to bring innovative drugs to the market has been complemented by an increasing use of diverse technologies such as wearables and mobile apps. Data has never been more accessible and the speed at which it is flowing in different forms and shapes, structured and unstructured, has left the industry reeling. Fundamental to the success of these advances, is the need to integrate data from diverse sources and leverage predictive analytics to drive informed, real-time decisions. To quote Nikhil Kumar, President, Applied Technol Solns Inc., “The “perfect storm” of medical science and technology has made data integration (DI) a critical success factor. New tools such as deep learning provide the foundation to the industry to do much more and see patterns faster in an automated fashion. This is providing further impetus to the rate of change and the criticality for innovative DI”. Pharma has recognized the criticality of DI and has been aggressively rethinking its DI strategy.
DI challenges – what can pharma do better?
今天的现实之一是缺乏立场ardization in pharma. An analysis conducted on six trials has demonstrated a variation of 20 to 50% in Case Report Form (CRF) elements, even within the same therapeutic area. Merely co-locating data and developing data schemas, table joins, aggregation logic, and output formats independently for each trial is neither an efficient nor a cost-effective approach. “The large volume and heterogeneity of data, high-dimensional data, hypothesis-driven data exploration and analysis, cross-platform data normalization, and data accessibility issues and regulatory restrictions,” pose further challenges according to Sagar Karmathi, Professor, Mechanical and Industrial Engineering and Director of Data Analytics Engineering Program, Northeastern University.
In fact, one study has demonstrated that the pharmaceutical industry spends $156 million annually on transferring data between systems or organizations. Further, point-to-point and ad hoc integrations can also impact data integrity as the possibility of errors is high. As pharma increasingly tries to leverage real world data by tapping eSource (electronic records documenting a patient’s health), it faces a roadblock with many EHR (Electronic Health Record)/EMR (Electronic Medical Record) vendors blocking the export of data out of their systems, posing more of a business challenge, rather than a technical one. Interoperability between the numerous systems and applications existing at pharma companies remains a nightmare.
Different generations of DI strategies
There has been a gradual evolution over the last few generations of DI strategy, starting with the use of relational databases, and SAS and SPSS, to data warehouses and data marts, awareness of the significance of master data management, the advent of noSQL databases, the explosion of big data, such as ‘omics’ data, and the advent of artificial intelligence (AI) and in particular machine learning (ML), and the cloud as a more mature, trusted platform. In addition, expert sourcing systems, unlike more primitive crowd sourcing systems, such as Tamr, are used to enable a hierarchy of internal and external business experts to participate in data curation decisions. Thus, DI models have evolved in terms of automation, scalability and architecture to deal with the increase in the volume, variety and velocity of data, balanced to some extent by a trade off on accuracy.
“Modern DI strategies are built on Hadoop-based data lakes and/or cloud-based data warehouses using data transformation tools like Spark and MapReduce. These DI strategies support high data velocities for information processing and system upgrades. They respond to enterprise needs in real-time through event-driven rather than clock-driven integration. They perform document-focused integration instead of raw-DI. They strive for inter-functional DI, flexible and adaptable DI, easy and any-time and any-where accessibility”, says Karmathi.
Big data, AI, ML: their role in pharma and DI
To be able to build scalable data curation systems we need to use tools such as ML, AI, big data and statistics. AI is used to simulate human intelligence such as visual perception and speech recognition and ML allows us to assess massive amounts of data simultaneously. As computing costs go down and the availability of data goes up, the doors to the use of precision medicine are opened. Despite possibly being able to cut down pharma costs by 70% there are still drawbacks of AI. These include the need to acquire reliable data sets, infallible, unbiased algorithms (not always the case!) and knowing which questions to ask (not something that AI can always do). Nevertheless, the benefits of AI arguably outweigh the negatives. According to Joseph Scheeren, senior advisor for R&D at Bayer, AI can potentially reduce the time required for a clinical trial by 30 to 40% and he rightly states that “In R&D, speed is everything”. Thus, the industry is gradually transitioning from the use of ePROs (electronic Patient Reported Outcomes) to eDROs (electronic Device Reported Outcomes), decreasing the dependency on patient perceptions of their own health.
话虽这么说,即使人工智能用于临床trials, it is designed to augment human intelligence rather than replace it. By using deep learning and AI, the industry is rapidly recruiting patients in clinical trials (especially for rare diseases) by mining social media and developing cures faster. AI could prove very useful in solving a lot of prevailing problems in the pharma industry, but it can only work effectively if it is asked the right questions. We can only truly optimize the healthcare industry if humans and machines work together.
“Everything we do in pharma/biotech is creating an enhanced experience for customers - Patients, Providers (Healthcare Professionals (HCPs), Healthcare Organizations and Payers. As competition grows, more generics and biologics are produced. The effectiveness in driving the efficacy of the therapies, the safety profile and the ease of use drives the unique value proposition of a particular therapy for pharma / biotech. This strategy has to be backed by connectedness with customers. To do all this, you need to understand customer sentiment, predict the likelihood of an adverse event, effectively understand the competition and its strategic moves ahead of time, evaluate HCP prescribing behaviors etc. This creates a need for big data analytics. Platforms such as Alexa are already working on AI with patient specific algorithms that can help with drug refill and infusion reminders. ML and deep learning are being used in accelerated disease identification / diagnosis, personalized treatment, accelerating drug discovery process, quicker mapping and grouping of target patients for clinical trials in big pharma to analyze large data sets and help in advancing FDA approvals” remarks Sai Vajha, Head, Enterprise Solutions and IT Operations, at Biogen.
Use cases for the application of DI in pharma and clinical trials
The potential value of effectively implementing DI in pharma is huge. It has been estimated by a medical device manufacturer that a reusable clinical data management platform would reduce efforts per trial by 75%, and creation time from one month to one week, translating into a 10-fold return on investment.
DI also serves as an enabler for the application of Bayesian statistics by empowering Bayesian statisticians to leverage intelligence acquired from other trials and to apply its enhanced predictive power to the analysis of the data of the current study. While this could both reduce the trial size and duration, care must be taken to determine how raw data from previous trials was identified and screened to remove significant "covariates." As a strong DI capability will allow for rapid cross-trial data analysis, this will reduce the number of data points required to statistically prove a product's safety and efficacy, reduce the number of subjects required, resulting in cost and time efficiencies. Since the average per-subject cost has been reported to be $2,500 per patient in drug trials and $10,000 per patient for device trials, a reduction in the number of subjects from 5000 to 3000 in a Phase III trial could potentially save a sponsor $5-$25 million.
The Data Manager to the Data Scientist
Drug development and data teams tend to work in silos. “Biomedical informaticians and clinical investigators often view each other as intellectual peasants providing rote/mechanical services.”, says Zak Kohane, Harvard in an interview with Forbes. But it takes two to tango and efficient, real-time coordination between clinical and data teams is crucial. Data management teams need to challenge their comfort zone, where they serve as data processors, and evolve into data scientists, where they draw meaningful insights from aggregated data sets and enable the clinical team to make informed, data driven decisions. ‘Understanding the math and understanding the tools are new skills that the Data Scientist should be trained on. Should we be awaiting the term “AI Scientist” as that field takes a foothold in the 5th generation of DI in pharma?”, wonders Kumar.
Data integration is playing a pivotal role in enabling pharma to trim its drug development strategy while accelerating innovation. Evolving roles and technologies are paving the way for data integration for the future.